Secure Agentic AI: Harnessing LLMs While Protecting Data Privacy
Enterprise telemetry is a goldmine of information, offering deep insights into system performance, reliability, and potential risks. But when it comes to leveraging the power of large language models (LLMs) for analyzing that telemetry, enterprises face a critical challenge: how to harness AI’s capabilities without exposing sensitive data.
The problem isn’t just about sharing raw logs or metrics. It’s about ensuring that every interaction with an LLM maintains the confidentiality and integrity of enterprise telemetry. Here’s why traditional approaches fall short and how IT teams can secure their data while unlocking the potential of advanced AI-driven insights.
The Risks of Raw Data Sharing
Sending raw telemetry data to an external LLM is a risky move that can expose your data—akin to handing your system’s keys to an unvetted contractor. Beyond the risk of data breaches, sharing raw logs can violate compliance regulations and expose proprietary information.
A Better Approach: Guided Analysis
Instead of feeding raw telemetry into an LLM, enterprises can flip the script. Rather than making the LLM process the data, let it guide what to look for. Here’s how this works:
- Keep the Telemetry Data Local: Enterprise telemetry stays within the organization’s infrastructure, untouched by external systems.
- Use LLMs for Context and Strategy: The LLM generates insights on what to search for, how to interpret patterns, or which correlations to explore.
- Leverage Internal Analysis: Based on the LLM’s guidance, internal tools and teams perform the actual analysis, ensuring sensitive data never leaves secure boundaries.
This approach turns the LLM into a powerful advisor rather than a direct processor of sensitive data.
Why RAG Alone Isn’t Enough
While RAG (Retrieval Augmented Generation) frameworks can filter and limit the data sent to an LLM, they still rely on external systems to interpret telemetry. This introduces potential vulnerabilities, as filtered data can still contain traces of sensitive information.
For example, a RAG-based system might expose a trend in authentication failures to an LLM, which could inadvertently highlight patterns about system usage or user behavior. These indirect insights can be just as risky as raw data.
By using LLMs as advisors instead of processors, enterprises eliminate this risk entirely. The model informs what to investigate, but the actual data never leaves the secure environment.
Real-World Example: Guided Root Cause Analysis
Imagine a team investigating recurring system crashes. Instead of sending logs to an LLM, they query it with a hypothetical: “What patterns in system logs typically indicate resource contention issues?”
The LLM provides guidance: “Look for overlapping spikes in CPU and memory usage over short intervals.” Armed with this insight, a secure AI agent searches for those patterns internally, keeping telemetry secure while benefiting from the LLM’s expertise.
The Future of Secure AI in Enterprise IT
As LLMs become more integrated into IT workflows, security must remain a top priority. Guided analysis represents a balanced approach—one that enables organizations to tap into advanced AI insights without compromising sensitive data.
At NeuBird, we’ve designed Hawkeye with these principles in mind, ensuring that enterprises can benefit from cutting-edge AI without sacrificing security. Hawkeye doesn’t just deliver insights—it collaborates with your teams, empowering them to make data-driven decisions while keeping telemetry safe.
If your organization is ready to explore how AI can securely transform IT security operations, schedule a demo today.
Written by
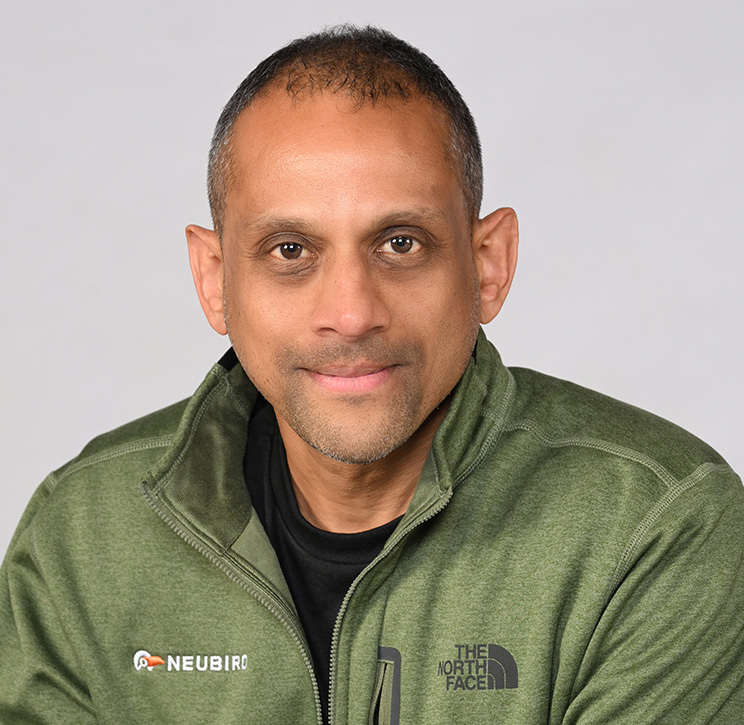